· news · 2 min read
Vironix Health continues its work with students on machine learning models to predict chronic kidney disease stages with high accuracy.
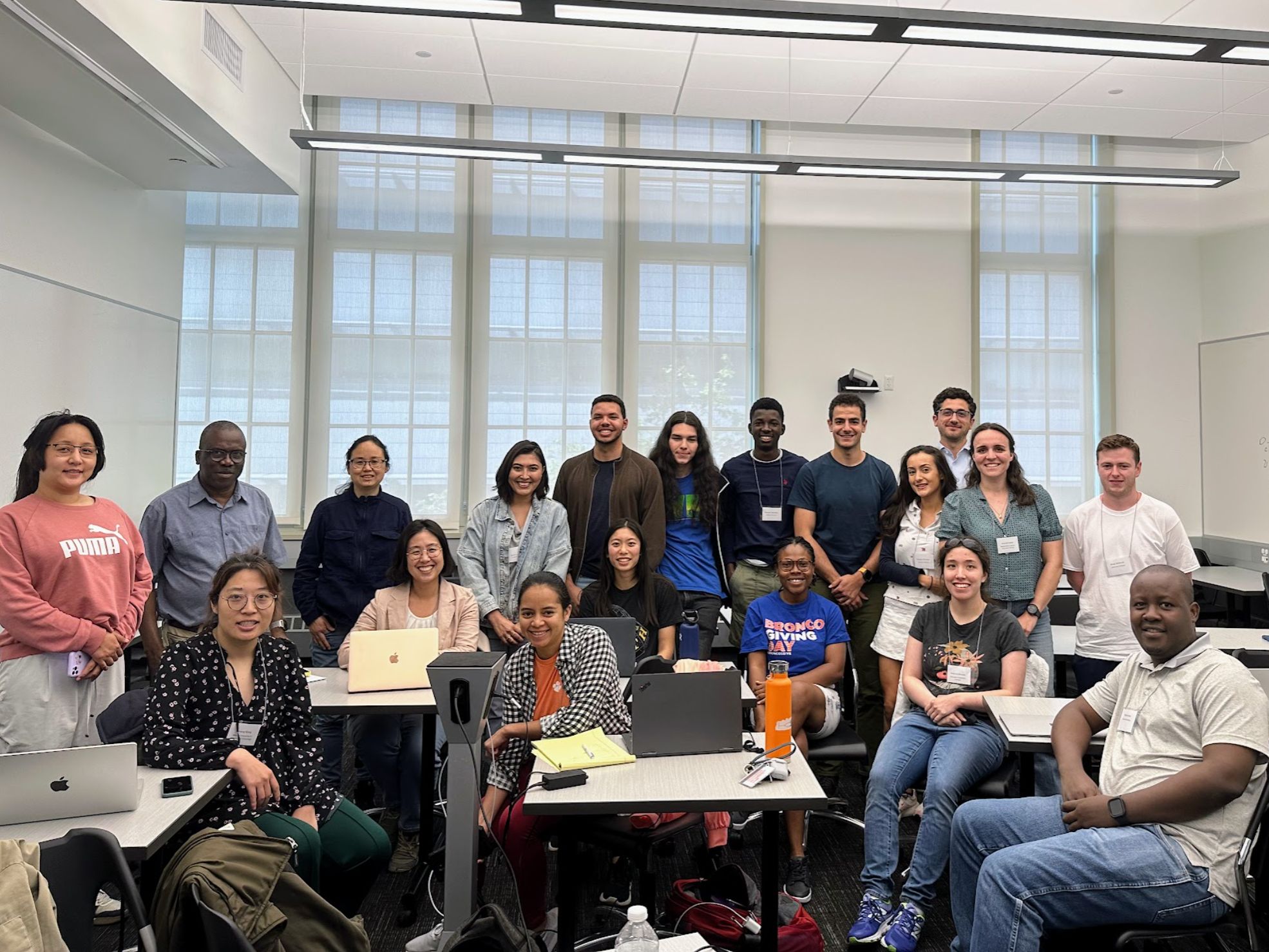
Vironix Health: Predictive Modeling for Chronic Kidney Disease (CKD)
Vironix was excited to continue its nearly 20-year involvement with students at the Society for Industrial and Applied Mathematics (SIAM) Math Problems in Industry Conference. From June 12 to 16, 2023, they worked on a groundbreaking project to develop predictive models and insights for managing CKD effectively.
By the end of the conference, the participants successfully identified a set of features, both generated at home and in the lab, that can indicate the deterioration of CKD. Through correlation analysis, they presented a list of features that showed a significant correlation with eGFR and creatinine levels. Using multiple machine learning models, they were able to classify the stages of CKD effectively. The best-performing model was a tree-based bagging model, achieving an overall accuracy of 77%. Notably, Stage 1 predictions achieved a remarkable accuracy of 98%, while Stage 3B had the lowest accuracy at 75%. The students’ work highlights the potential of using such models for accurate stage classification and risk assessment in CKD.
The need:
📚 Chronic Kidney Disease (CKD) Progression: CKD patients experience a gradual and often silent decline in kidney function, necessitating intensive management and treatment.
💡 Early Intervention: Identifying CKD patients requiring intensified care and personalized treatments is crucial for better outcomes.
💻 Predictive Models: Cutting-edge predictive models can play a pivotal role in identifying patients in need of more aggressive management and providing valuable insights for outpatient screening.
Project Goals: Data Identification: Identify representative data from scientific literature and public databases to capture the clinical characteristics of CKD patients. Vironix provided a publicly available dataset to kickstart the project. Methodology Development: Determine an effective methodology for using the identified data to predict severe/non-severe presentations of CKD. Explore various approaches, including those beyond our current expertise. Model Development and Validation: Develop and validate a prediction model with reasonable accuracy, sensitivity, and specificity for CKD patients. Clinical Feature Identification: Highlight and describe the most critical clinical features relevant to accurately predict CKD patients.
Synthetic Data Evaluation: Generate synthetic data from the original dataset and assess the performance gains achieved when training and testing prediction models using both synthetic and real data.